There is huge appetite and excitement for having greater intelligence at the edge to drive insights from data where it is created. A recent study sponsored by Microsoft has revealed that 79% of enterprises currently have or will have some form of edge compute strategy. Companies are increasingly turning to the edge as a way to take the strain off their networks, make use of new service offerings and lower the costs of connecting to traditional data centres. In fact, the strategy has become so popular that Gartner predicts that by 2025 more than 50% of enterprise-managed data will be created outside of the data centre or cloud[1].
Edge is a trend that enterprises of all types are looking to build expertise in and companies are racing to develop a strategy that aligns to their move toward digitalisation. Clarity has been lost as studies and consortia seek to define edge in their own terms and according to their own market positions. The hype is running ahead of reality and the more people try to compartmentalise or define edge, the wider it becomes.
Building an edge strategy is not a sprint but a marathon
The narrative about sprinting towards the edge underscores that edge is urgently needed to improve operational performance and control costs. However, edge transformation will be more of a marathon than a short-term sprint. A narrow focus on speed, connection density and low latency, as envisioned in the hype surrounding technologies like 5G, doesn’t address how network infrastructure needs to evolve to support the IT-focused platforms and as-a-service business models that enterprises want to introduce or leverage.
Organisations will need to re-assess such critical topics as data governance, information retention and usage policies. Handling the radically increasing amount of data – IDC foresees 79 zettabytes of data being created by billions of IoT devices by 2025 – must be enhanced and streamlined.
Memory unlocks edge data value
Growth in hardware infrastructure will be driven by on-premise, AI-accelerated, low-latency systems that host edge-based platforms. However, either by hosting or aggregating streams of data, edge computing will rely on the edge appliances’ memory and storage to populate and feed applications such as artificial intelligence, predictive maintenance and other use cases.
Demands on memory technology have also increased with the growing number of CPU with multiple cores and the pressure to match next-generation bandwidth requirements. These complex systems require faster computing and automated decision-making.
However, memory bandwidth per core has been a bottleneck for faster compute solutions and so, the typical solution will be to add more dynamic RAM (DRAM) to accommodate the compute need.
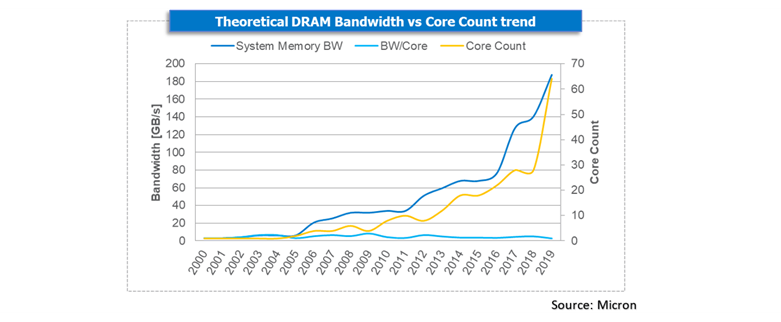
Edge compute systems that need to a support machine learning inference up to GPU-level compute performance, will require high performance DRAM solutions, not just in terms of megatransfers per second (MT/s) throughput but incorporate more efficient memory bank usage and therefore improve overall effective bandwidth. For example, Micron compared our DDR4 at an equivalent data rate of 3200 MT/s vs our latest DDR5 system-level simulation which indicates an approximate performance increase of 1.36x effective bandwidth. At a higher data rate, DDR5-4800 will have an approximate performance increase of 1.87x – nearly double the bandwidth as compared to DDR4-3200.
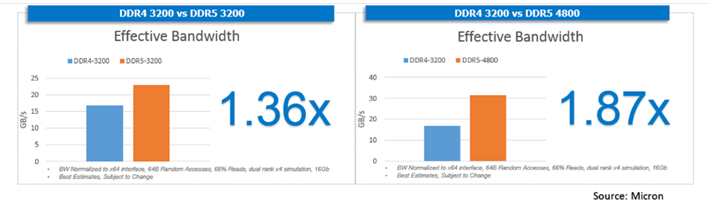
With the understanding that memory along with storage and IT-readiness are essential elements of a successful edge strategy, implementation becomes less about speed and more about setting a steady, methodical pace to ensure goals are met.
To learn how memory is fuelling digital transformation by enabling IoT applications at the edge, visit: www.micron.com/solutions/industrial-iot
The author of this blog is David Henderson, Director of Segment Marketing, Embedded Business Unit and Micron Technology
[1] https://www.channelfutures.com/from-the-industry/the-future-of-edge-computing-is-changing